At the prestigious New York Learning Hub, Engineer Samuel Lawrence, a distinguished researcher, software engineer, and intelligence officer with the Nigerian Police, recently presented groundbreaking research on the transformative impact of Artificial Intelligence (AI) on business process optimization. His study, titled “AI-Driven Business Process Optimization: Enhancing Operational Efficiency in Management,” provides a comprehensive exploration of…
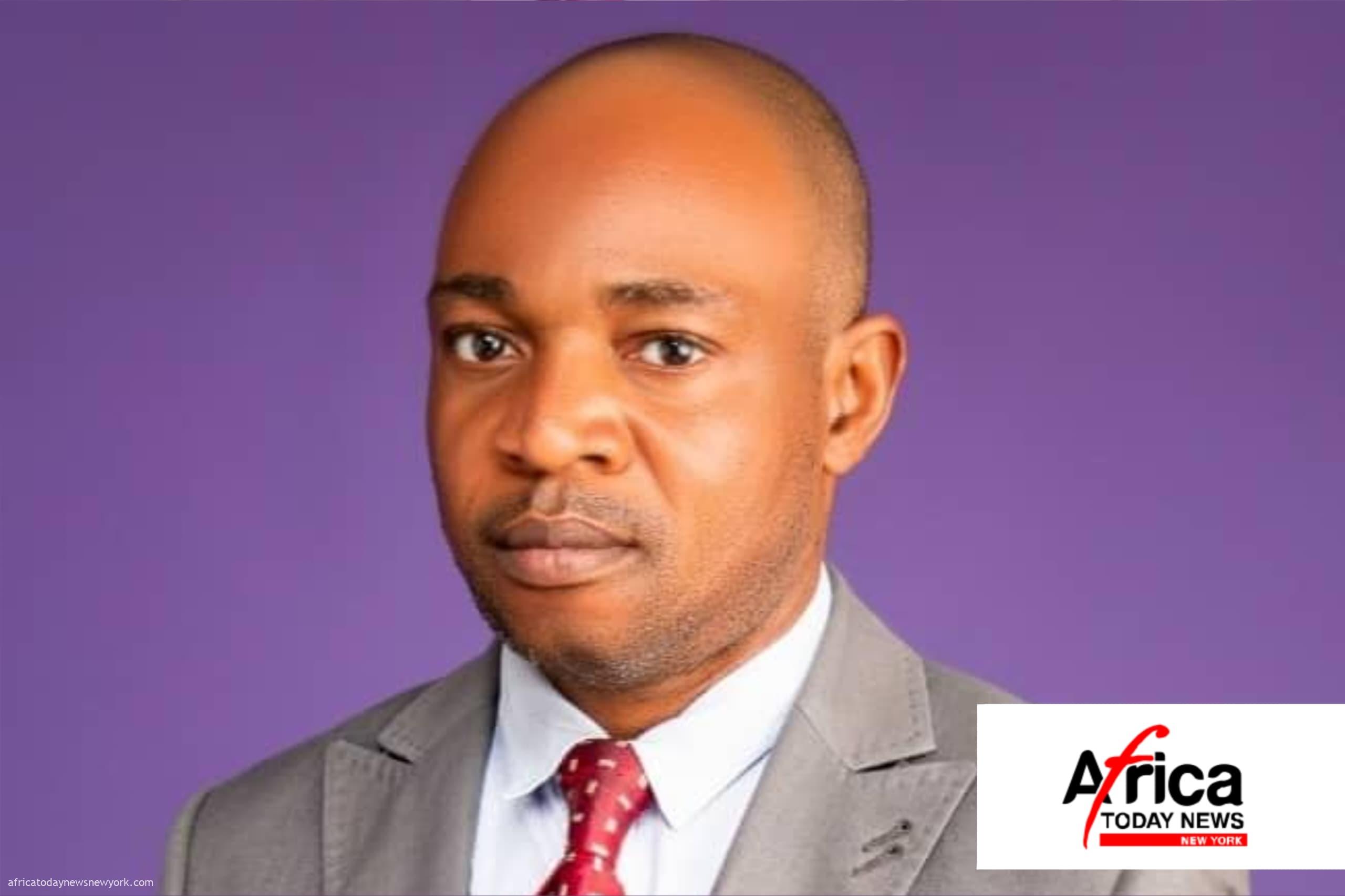